Navigating Market-Product Fit, Pricing Tiers, and Emerging AI Trends: Lessons from Brian Balfour, OpenAI, and Pictotales
In the indie hacker world, we often emphasize building in public—openly sharing our progress, experiments, and even our struggles. Today, I’d like to do exactly that by analyzing Brian Balfour’s Market-Product Fit theory, reflecting on the logic (or lack thereof) behind OpenAI’s pricing tiers, and exploring where my own project, Pictotales, has faced pricing missteps. My hope is that this post offers concise yet meaningful insights for creators, founders, and innovators.
1. Brian Balfour’s Market-Product Fit in a Nutshell
Brian Balfour is known for reframing the concept of “Product-Market Fit” to put the market first—rather than the product. Below is a brief refresher on his key points:
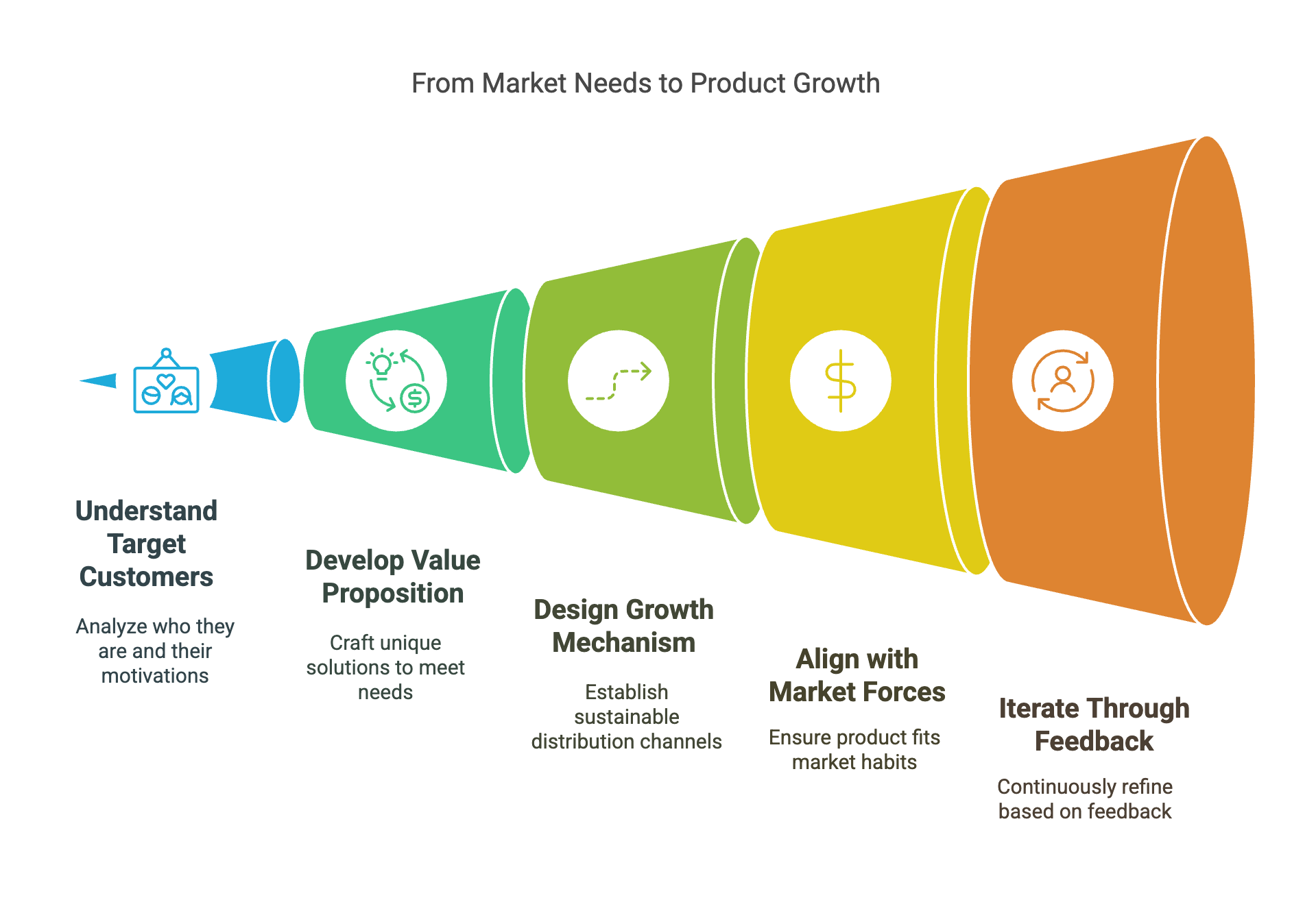
- Market Comes First
Instead of building a product and then hunting for a market, identify a target audience with urgent pain points or strong desires. This ensures that the market itself “pulls” the product into existence. - Market-Product Fit > Product-Market Fit
Balfour flips the order—by the time you build, you already know the market’s needs. In other words, market demand helps shape the product so it resonates more powerfully. - Three Pillars of Market-Product Fit
- Target Customers: Who they are, what they care about, and why.
- Value Proposition: How you solve their critical pain or cater to a strong desire, in a unique way.
- Growth Mechanism: How your product naturally grows within that market (i.e., the distribution channels and loops that sustain it).
- Distribution and Market Forces > Product
Even a brilliant product can flounder if it doesn’t align with existing market habits, distribution channels, and customers’ willingness to pay. - Iteration Through Feedback Loops
You never hit perfect market fit on your first try. Continuous iteration—collecting data, refining, repositioning—is essential.
Applying these principles is crucial in any SaaS or AI service. They also give us a helpful lens through which to evaluate evolving business models, like OpenAI’s pricing structure and my own experiences with Pictotales.
2. Examining OpenAI’s Pricing Model
OpenAI currently offers three subscription tiers for ChatGPT: Free, Plus, and Pro.
- Free Plan
- Basic ChatGPT features, using the GPT-4o mini model.
- Unlimited messages on web, iOS, and Android.
- Plus Plan at $20/month
- All Free Plan features.
- Unlimited access to GPT-4o mini, limited access to GPT-4o.
- Limited advanced tools (like DALL·E, web browsing, data analysis).
- Standard voice mode and custom GPT creation.
- Pro Plan at $200/month
- Unlimited access to GPT-4o, GPT-4o mini, and voice.
- Higher limits for video/screensharing in voice.
- o1 pro mode: a higher compute setting for more complex queries.
- Extended features such as Sora video generation and early Operator research previews (U.S. only).
2.1. Potential Disconnect from Market-Product Fit
Despite OpenAI’s impressive capabilities, there’s a noticeable pricing chasm: from $20/month to $200/month. The jump is significant, and some users are left wanting a middle ground for advanced features—like deep research agents or heavy compute—without paying $200.
Balfour’s focus on Market-Product Fit suggests you need clear alignment with how each customer segment values your product. Right now, OpenAI’s distribution of features (especially the agent-based “Operator” or deep research tools) is restricted to the Pro tier, potentially misaligning price-to-feature for many advanced but not enterprise-level users. Could a $50–$70 tier fill this gap and capture more mid-market professionals or small startups? Possibly.
3. Why No Middle Price Tier?
It’s natural to wonder why OpenAI hasn’t introduced a $50–$70 “Advanced” tier. Below are a few hypotheses, tying back to Balfour’s market-first approach and broader AI business strategy:
- Cost Structure & Scalability
Deep research agents and advanced model usage incur high compute costs. If a large portion of the user base opts for these computationally expensive tools at $50–$70, it might pressure margins. The gap in the price tiers could reflect internal cost analyses where such a middle tier might become a loss leader or threaten the profitability of the Pro plan. - Focus on Premium, Enterprise-Level Buyers
The $200 Pro tier might be aimed at enterprise professionals, teams, or power users with specific budgets. By having a steep jump, OpenAI might be implicitly segmenting those who really need advanced features versus casual or semi-professional users. - Bundling & Brand Positioning
A simpler tier structure can avoid confusion. By offering only three tiers, they maintain clarity. Also, “Pro” can remain an exclusive label, preserving brand prestige. Meanwhile, the gap might be bridging soon (or might not) depending on internal R&D and data. - Forward-Looking AI Cost Trends
Some believe that generative AI cost curves will continue to fall, thanks to scaling laws, Model of Experts (MoE), knowledge distillation, or better GPU efficiency. OpenAI may be betting on future cost reductions that will allow them to keep—and eventually expand—these tiers without introducing a middle tier right now. - Caching & Reuse
OpenAI could plan on caching prompts, reusing certain computations. If a majority of user queries are repetitive, they could offset deep research costs through intelligent caching, effectively lowering the cost of advanced usage for the $20 tier eventually. But it’s not guaranteed to be a perfect solution at this stage. - Strategic Bet on User-Generated Data
Unless OpenAI believes that offering deep research capabilities at $20 might produce synthetic data whose long-term value surpasses incremental revenue from higher ARPA (Average Revenue Per Account), they have little incentive to open that feature to lower-tier customers. If that data—generated by a massive user base—proves more beneficial for model training and future product improvements, it could theoretically justify including deep research in the $20 plan. Otherwise, pushing that feature to higher tiers helps preserve margins and maintain premium exclusivity.
4. Lessons from Pictotales: When Pricing Goes Awry
I’ve wrestled with similar dilemmas in my own project, Pictotales, which uses AI to generate illustrated picture books and stories. Our pricing tiers were:
- Free – A small daily story allowance, letting people try it out.
- Standard ($15/month) – 4 StoryPoints monthly, AI story generation, and library access.
- Premium ($25/month)– 10 StoryPoints monthly, audio generation, advanced library, and early access to new features.
4.1. Why It Didn’t Work Well
- Image Generation Is Expensive
AI image generation can be more costly than text generation, especially at scale. Audio generation also adds to the compute burden. This meant our cost-per-user soared whenever they generated a large number of picture books or audio content. - User Value Perception
Subscribers wanted an ever-increasing range of story types, illustrations, or custom voice narrations—raising costs even more. If we priced high enough to cover the cost, it became unaffordable for many parents. If we kept it cheap, we faced margin pressure. - Market-Product Fit Issues
We built the product around the potential for AI-illustrated stories but only belatedly validated whether parents were willing to pay at scale. There was a mismatch in how we priced features versus how the market valued them.
5. Rethinking Pricing and Feature Bundling
Considering Balfour’s approach, it’s best to let the market guide the product design and pricing. For OpenAI, a new $50–$70 tier might allow:
- Deep Research Lite with some compute limitations.
- Extra Collaboration Tools suitable for small teams or advanced freelancers.
- Partial Sora or Operator Access in limited capacity, rather than fully locked behind $200.
The argument is that a mid-tier helps capture a significant slice of the market that’s advanced enough for serious usage but not enterprise-scale. It also could reduce the sticker shock that might push high-volume enthusiasts to churn or remain on $20 while feeling underserviced.
5.1. Counterarguments and Caveats
- Cost of Deep Research: Deep research agents might still be too expensive at scale. Even at $50–$70, many users conducting heavy research would drive up costs. OpenAI might risk subsidizing them or introducing usage caps that complicate the plan.
- Brand Erosion: If exclusive features trickle down to a mid-tier, the $200 plan might lose some of its premium aura. Power users paying $200 might downgrade if they get most of what they want at $50–$70.
- Clarity vs. Complexity: Introducing more tiers can confuse users and complicate marketing. Each new plan has to be carefully positioned to keep sign-up flows simple.
6. A Self-Critique of Pricing Strategies (and Moving Forward)
Reflecting on Pictotales with a clear vision of helping busy parents create interactive, memorable stories alongside their children, I’ve come to see both the strengths and shortcomings of our current pricing model:
- Bundling a Library
Offering a ready-made library of AI-generated picture books was a strategic move. It shifts part of the product’s value from on-demand generation to easily accessed, “already done” content. This approach helps justify a higher subscription price for parents who want a quick, frictionless way to spark storytime with their kids—no fuss, no wait. - Audio Generation: A Premium Hook
Adding voice narration and music can be magical for children. However, audio generation is computationally costly. If included in lower-priced tiers, it risks cannibalizing profits. Conversely, keeping it locked behind a pricier subscription can frustrate parents if they’re unclear on why it costs so much. Striking the right balance—highlighting the unique value of audio narration while covering the costs—is essential. - Encouraging Parents to Upgrade
The biggest challenge has been whether the leap from our free or standard plan to the higher-priced premium tier truly feels worthwhile for busy parents. If the jump in price overshadows the perceived benefit, parents won’t make the switch. Conversely, if the price gap is too small, we may lose margin.
6.1. Toward a Better Solution
- Highlight Emotional Value
Parents are often willing to invest if they see clear, immediate benefits. Emphasizing the emotional bond created during storytime—enhanced by richer features like illustrated stories, personalized narratives, or audio narration—makes the upgrade feel indispensable. - Optimize Tier Gaps
A well-calibrated pricing ladder ensures each step up feels logical. Maybe the free tier grants a limited taste (a few story generations), the mid tier satisfies casual use, and the premium tier offers a clear “wow” factor—unlocked library access, audio narration, and curated story bundles. - Usage-Based Add-Ons
Instead of including expensive features in the standard plan, we could offer à la carte upgrades (e.g., pay-per-use audio enhancements) or bundle them into a premium “family pack.” This way, cost-intensive features don’t sink our margins, yet parents who truly want premium interactions can easily opt in. - Iterative Testing
Pricing is never final. Conducting short-term experiments (e.g., offering parents a week of premium features for free to gauge usage and satisfaction) can reveal what truly motivates an upgrade. Collecting usage data and feedback directly from parents—the real decision-makers—helps refine the tiers over time.
Ultimately, the key is to remain aligned with what busy parents want and what they’re willing to pay for. By combining a compelling value proposition (memorable story sessions with kids) and a pricing structure that sensibly differentiates each tier, we can nudge more families to embrace the premium plan—without sacrificing sustainability.
7. Conclusion
When shaping or reshaping any AI-based product:
- Validate the Market First: Who exactly needs advanced features? How much are they willing to pay?
- Align Features with Value: Make sure each tier resonates with the right segment’s willingness to pay and usage patterns.
- Iterate Fearlessly: Product-market dynamics shift rapidly in AI. Keep testing, learning, and tweaking.
OpenAI’s steep pricing jump, my own misadventures in pricing Pictotales, and Brian Balfour’s Market-Product Fit framework all point to the same overarching conclusion: Pricing is never static. It’s a dance between product capabilities, user demand, cost structure, and brand strategy. As indie hackers building in public, we have the advantage of being able to pivot quickly—let’s use that agility to our advantage, leveraging market signals to create better fits for our users and our own business goals.
Thanks for reading! If you have insights or experiences with tiered AI pricing or want to swap notes on building in public, feel free to reach out or leave a comment. I’d love to continue the conversation on how we can refine both product and pricing strategies to meet the evolving demands of AI-driven markets.